One of the most difficult aspects of X-Ray microscopy is balancing throughput with picture quality. Long acquisition times are required to obtain high-quality pictures, especially when working with big samples. The technology’s extensive acquisition times may limit its use in industrial operations where sample time is crucial, or in situ investigations where great temporal precision is required.
Through the incorporation of neural networks into the image acquisition and reconstruction process, ZEISS DeepRecon allows for dramatic gains in image quality and effective acquisition time. Networks and procedures can be tailored to address unique difficulties associated with repeating samples by collaborating with clients.
This technique has the potential to boost throughput by a factor of 10, improve picture quality and lessen the influence of imaging artifacts, which are common in rapid acquisition workflows.
Highlights
Area of Research
- Geoscience
- Manufacturing
- Semiconductor
Sample Types
- Semiconductor packages
- Geological samples
- Electronics
Related Solutions
- ZEISS Phase Contrast Enhancer
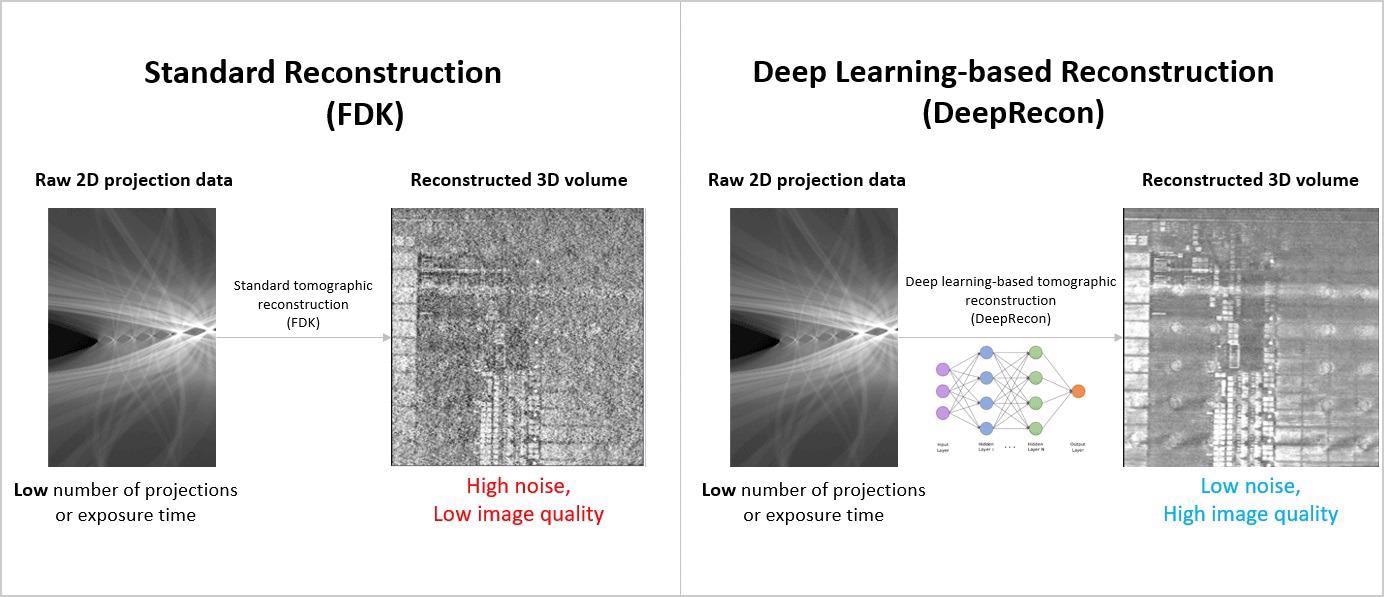
Deep learning-based image quality improvement with higher throughput for Xradia X-ray microscopes. Image Credit: Carl Zeiss Microscopy GmbH
Training neural networks to recover image data damaged by noise, restricted projection numbers or samples outside of the field of vision is the structure of neural network-based picture quality enhancement.
Workflow
The Module Workflow Consists of Several Steps
- ZEISS DeepRecon is a Special Customer Solution (SCS) that is tailored to the use-case and sample class’s individual needs. To begin the conversation about implementing DeepRecon at their facility, contact the local ZEISS salesperson (or fill out the form below)
- Send a sample of data to the ZEISS XRM team
- This information is utilized to develop a customized DeepRecon model, which allows for better results
- This model is imported into a ZEISS Reconstructor system that has been modified
- Gather information under the conditions mentioned (up to 10X throughput boost relative to standard reconstruction). During sample reconstruction, the customized model will be an available option
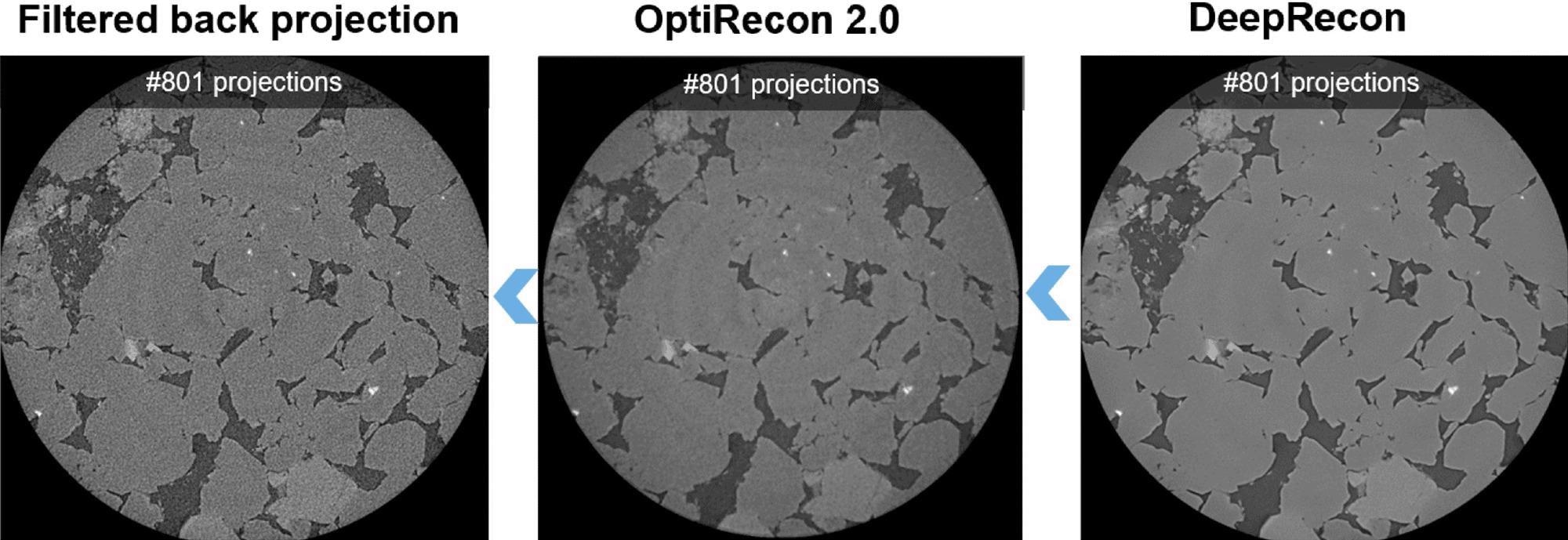
Progressive image quality improvement with various reconstruction techniques for Xradia X-Ray microscopes. Image Credit: Carl Zeiss Microscopy GmbH
The above image shows the impact of several reconstruction approaches on a sandstone sample, demonstrating progressive picture quality improvement from conventional reconstruction (filtered back projection) through OptiRecon 2.0 (iterative reconstruction) and DeepRecon 2.0 (deep reconstruction) (neural network-based reconstruction).
Required Components
- ZEISS DeepRecon reconstruction technology
- ZEISS Xradia Versa XRM